In this interview, HPI alumnus David Jaeger provides insights into the development of the HPI Identity Leak Checker since the project launch in 2014.
>
Go to article
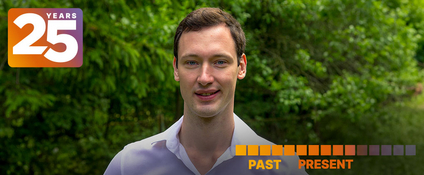
Learn more about how artificial intelligence can help make the world more sustainable.
>
Go to article
