Hack den Sommer mit deinen Ideen im HPI-Sommercamp 2024! Fünf Tage lang tauchst du mit anderen Jugendlichen in die Welt der Informatik ein.
>
Zum Artikel
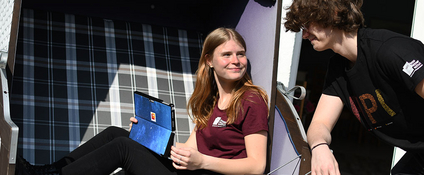
Beim Young Women in AI Camp lernen Schülerinnen vom 16.-18. Oktober die Welt der künstlichen Intelligenz kennen.
>
Zum Artikel
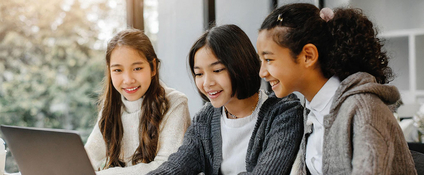
Die empowerHER+ Konferenz des HPI schafft Raum für die aktuellsten IT-Themen sowie Vernetzung und Empowerment von Frauen* in der Tech-Branche.
>
Zum Artikel
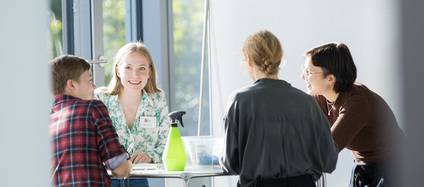
Die ganze Nacht wach bleiben und coden: Das geht für alle IT-Begeisterten ab 16 Jahren zur HPI Code Night im November.
>
Zum Artikel
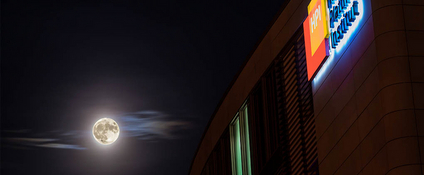