Zum Girls'Day am HPI in Potsdam lernen Schülerinnen, einen Minicomputer zu programmieren und warum das Informatikstudium so vielfältig ist.
>
Zum Artikel

Die HPI Connect Messe am 16. Mai 2024 bringt Firmen aller Branchen mit HPI-Studierenden und -Alumni zusammen.
>
Zum Artikel
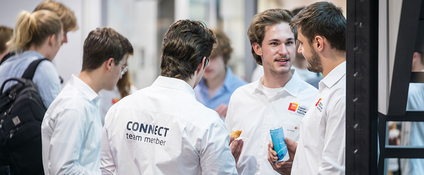
Nach dem Abitur die digitale Zukunft gestalten: Informiere Dich am 07. Juni über den Bachelor IT-Systems Engineering.
>
Zum Artikel
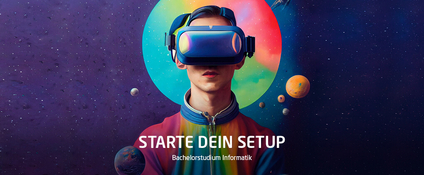
Im Girls@BwInf Camp vom 13.-16. Juni entwickeln die Schülerinnen in kleinen Teams eigene Smart-Home-Lösungen.
>
Zum Artikel
