Die HPI Connect Messe am 16. Mai 2024 bringt Firmen aller Branchen mit HPI-Studierenden und -Alumni zusammen.
>
Zum Artikel
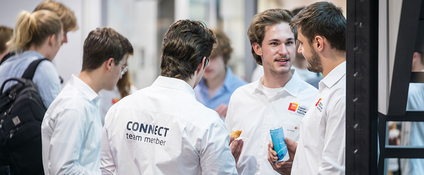
Nach dem Abitur die digitale Zukunft gestalten: Informiere Dich am 07. Juni über den Bachelor IT-Systems Engineering.
>
Zum Artikel
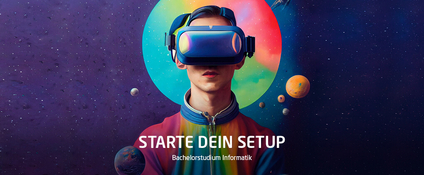
Im Girls@BwInf Camp vom 13.-16. Juni entwickeln die Schülerinnen in kleinen Teams eigene Smart-Home-Lösungen.
>
Zum Artikel

Am 19. und 20. Juni 2024 diskutieren hochrangige Expert:innen am HPI über die aktuelle Lage der Cybersicherheit in Deutschland und der Welt.
>
Zum Artikel
